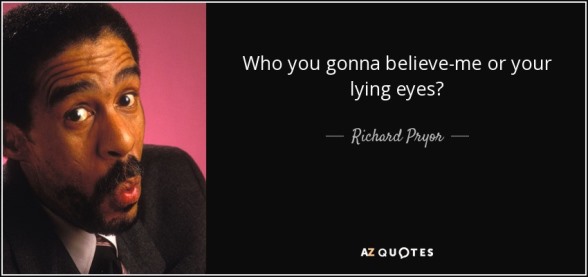
Our work on machine learning to predict suicide attempts and suicide deaths is moving rapidly to implementation. Among mental health specialty visits, we are now able to accurately identify the 5% of patients with a 5% likelihood of a treated suicide attempt in the following 90 days. Our health system partners believe those predictions are accurate enough to be actionable. For example, clinicians might be expected to conduct and record a structured suicide risk assessment in any visit with a predicted risk of greater than 5%. And clinics or health systems might be expected to reach out more assertively when a high-risk patient cancels or fails to attend a mental health appointment. With interventions like this in mind, mental health leaders at Kaiser Permanente Washington are planning to import computed risk scores into our electronic health record. We will use those predictions to prompt systematic risk assessment and outreach in our mental health specialty clinics. We expect that other MHRN health systems will soon follow.
As MHRN researchers have discussed this implementation with mental health system leaders, one question comes up over and over: How should we integrate risk predictions based on machine learning models with risk predictions based on direct clinical assessment? In many of our health systems, clinicians use responses to Item 9 of the PHQ9 (regarding thoughts of death or self-harm) to identify patients in need of additional risk assessment. This standard work is now built into many of our electronic health records systems.
We have no doubt that machine learning algorithms outperform our current clinical assessment tools. Among mental health specialty visits, a response of “more than half the days” or “nearly every day” to Item 9 of the PHQ9 identifies approximately 6% of visits. That group has a 2% likelihood of treated suicide attempt in the following 90 days. This compares to a 5% likelihood following visits with risk scores in the top 5%. No matter where we set the threshold, machine learning risk scores are both more sensitive (i.e. miss fewer subsequent suicide attempts) and more efficient (i.e. accurately predict a large number of suicide attempts in a smaller number of people).
Nevertheless, it’s difficult to advise providers to now ignore those PHQ9 responses regarding thoughts of death or self-harm. Our mental health leaders have invested significant time and energy in training providers to respond systematically to self-reported risk. Reversing course now certainly could be confusing. Our clinicians might wonder how an algorithm could outperform a face-to-face conversation. Out in the wider world, trust in algorithms and big data is not having a good year.
I jokingly refer to this as “The Richard Pryor Problem,” after the quote sometimes attributed to the comedian Richard Pryor and sometimes to an even older comedian, Chico Marx: “Are you going to believe me, or your own lying eyes?” In each case, that punch line is delivered by a cheating man hoping to confuse and undermine his suspicious partner. That makes for an uncomfortable analogy to our suggestion that clinicians believe an algorithm over direct observation.
But the analogy does not fit in one important respect. Our suicide risk prediction models depend entirely on what clinicians have seen with their own eyes. The strongest predictors of suicide attempts and suicide deaths include prior mental health diagnoses and mental health treatments determined by treating clinicians in traditional clinical encounters. Our algorithms do not discover anything that treating clinicians could not see; they simply average across millions of clinical judgments to make more reliable predictions.
For now, our solution to the Richard Pryor Problem is a compromise. When we import risk prediction scores in the electronic health records, we will advise providers to use structured risk assessment tools whenever a patient has a computed risk score in the top 5% OR directly reports frequent thoughts of death or self-harm on the PHQ9. In other words: You should believe the algorithm, but you don’t have to ignore what your own eyes are telling you.
Greg Simon