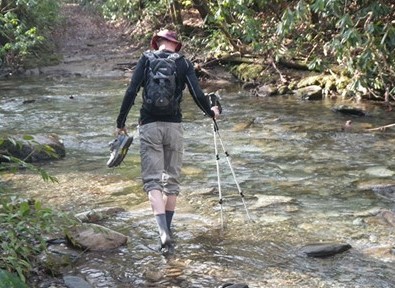
Our randomized trial of outreach programs to prevent suicide attempts tested a long step beyond what we knew from previous research. We hoped that low-intensity adaptations of proven effective interventions – delivered primarily online – could scale up to prevent suicide attempts at the population level. And we were wrong. Not only did neither of the programs we tested prevent self-harm or suicide attempts, one of them may have increased risk.
Looking back, we can say that we tried too long a step beyond interventions proven to work. We can try to unpack that long step into a few smaller pieces. First, our trial included the broad population of people at increased risk (where most suicide attempts occur) rather than the much smaller population of people at highest risk (where previous interventions had been tested). Second, we emphasized outreach to people who were not seeking additional help rather than limiting to volunteers who agreed in advance to accept the extra services we were offering. Third, we tested low-intensity interventions, delivered primarily by online messaging rather than more personal and intensive interventions delivered by telephone or face-to-face.
We could have started by separately testing each of those smaller steps rather than trying to cross the creek all at once. But any smaller trial testing one of those small steps would have taken two or three years. Testing one smaller step after another would have taken even longer. Given rising suicide mortality rates throughout the 2010s, we chose not to wait several years before trying a large step. We believed the programs we tested were close enough to the solid ground of proven interventions, and we certainly hoped they would expand the reach of effective prevention.
Regardless of the time required, it may not have been helpful to divide that big step into smaller pieces. We could have limited the trial to people at highest risk, but then we would not have studied low-intensity online interventions. We could have limited the trial to people who agreed in advance to accept extra services, but then we would not have studied outreach interventions.
After we published our findings, we did hear questions and suggestions about each piece of the big step we tried: Why not focus on those at highest risk? Why not test more intense or robust interventions? Why include people who were not interested in the treatments you were offering? But if we’d done all of those things, we would have just replicated research that was already done – and ended up right back where we started. Back in 2015, we already knew that traditional clinical interventions, like Dialectical Behavior Therapy, could decrease risk in treatment-seeking people with recent self-harm or hospitalization. Replicating that evidence would not inform population-based prevention programs for the broader population of people at increased risk.
We are certainly not giving up on the idea of population-based programs to prevent suicidal behavior. So we’re thinking about ways to try smaller steps. Rather than small steps, we may need to look for a completely new place to get across the creek. Our suggestion box is open.
Greg Simon