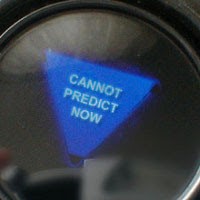
This post will be a nerdy one. I want to split some hairs about use of the word “predict”. But I think they are hairs worth splitting.
By the dictionary, prediction is always about the future. But we researchers sometimes use the word more loosely. We might say that “A is a significant predictor of B” even when A and B occur at the same time. For example, an evaluation of screening to identify suicidal ideation might report how well screening questions “predict” risk assessed by an expert clinician during the same visit. That’s really an example of detection or diagnosis rather than prediction. But I don’t expect that my hair-splitting will end use of the word “predict” to describe what’s really a cross-sectional association or correlation. It’s a longstanding practice, and saying that “A predicts B” just sounds more important than “A is associated with B.” Still we should remember Yogi Berra’s point that predicting the future is a very different task from predicting the present.
Yogi’s wisdom is especially relevant to our interpretation of Positive Predictive Value or PPV. Ironically, the concept of PPV was originally developed for a scenario that is not, by the dictionary definition, prediction. Instead, PPV was intended to measure the performance of a screening or diagnostic test. Traditionally, PPV is defined as the proportion with a positive test result who actually have the condition of interest – not the proportion who will develop that condition at some point in the future. The PPV metric, however, can also be used to measure the performance of a prediction tool. Those are two very different tasks, and our thresholds for acceptable PPV would be quite different. Consider two examples from cardiovascular disease. If we are evaluating the accuracy of a rapid troponin test to identify myocardial infarction (MI) in emergency department patients with chest pain, we’d expect that most patients with a positive troponin test to have an MI by a confirmatory or “gold standard” test. But if we are using a Framingham score to predict future MI, we would not require nearly so high a PPV before recommending treatment to reduce risk. In fact, most guidelines recommend cholesterol-lowering medications when predicted 10-year risk of MI is over 10%.
The utility of any PPV depends in part on overall prevalence. If 25% of emergency department patients with chest pain are having an MI, then a PPV of 30% is hardly better than chance. But if the average risk of MI is only 1%, then a PPV of 10% could be quite useful. And our threshold for PPV also depends on the intervention we might recommend. We’d likely require a higher PPV before recommending angioplasty than we would for recommending statins. Predictions about the future more often involve rarer events and less intensive interventions.
I think that confusion about these different uses of PPV has led to exaggerated pessimism regarding tools to predict suicidal behavior. In that case, our goal is actual prediction – predicting a future event rather than detecting or diagnosing something already present. Current prediction models can accurately identify people with a 5% or 10% risk of suicide attempt within 90 days. That would be a poor performance for a diagnostic test, but it’s certainly good enough actual prediction. A 5% risk over 90 days is a much higher risk threshold than we use to prescribe statins to prevent MI or even anticoagulants to prevent stroke in atrial fibrillation.
Translating Yogi Berra’s wisdom into technical terms, we might say: “We would accept a much lower PPV for a prediction model than we would for a diagnostic test.”
What about that other famous Yogi statistical quote: “Ninety percent of the game is half mental.” Was he trying to explain the difference between mean and median in a skewed distribution? You never know…
Greg Simon