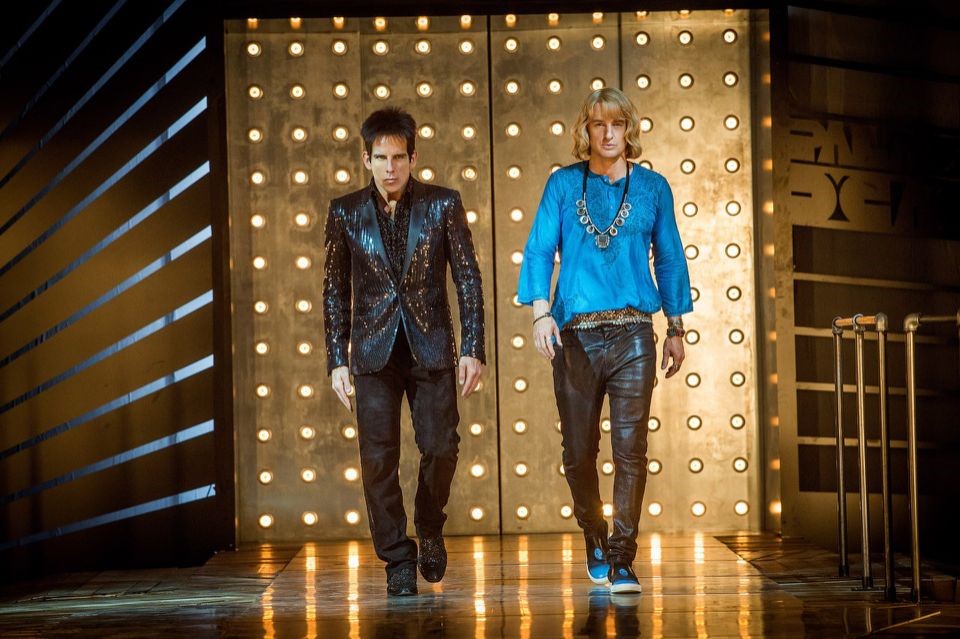
Projections of the course of the COVID-19 pandemic prompted vigorous arguments about which disease model performed the best. As competing models delivered divergent predictions of morbidity and mortality, arguments in the epidemiology and medical twitterverse grew as rowdy as the crowd in that male model walk-off from the movie Zoolander. Given the rowdy disagreement among experts, how can we evaluate the accuracy of competing models yielding divergent predictions? With all respect to the memory of David Bowie (the judge of that movie modeling competition), isn’t there some objective way of judging model performance? I think that question leads to a key distinction between types of mathematical models.
Most models predicting individual-level events (such as our MHRN models predicting suicidal behavior) follow an empirical or inductive approach. An inductive model begins with “big data”, usually including a large number of events and a large number of potential predictors. The data then tell us which predictors are useful and how much weight each should be given. Theory or judgment may be involved in assembling the original data, but the data then make the key decisions. Regardless of our opinions about what predictors might matter, the data dictate what predictors actually matter.
In contrast, models predicting population-level change (including many competing models of COVID-19 morbidity and mortality) often follow a mechanistic or deductive approach. A deductive model assumes a mechanism of the underlying process, such as the susceptible-infected-recovered model of infectious disease epidemics. We then attempt to estimate key rates or probabilities in that mechanistic model, such as the now-famous reproduction number or R0 for COVID-19. “Mass action” models apply those rates or probabilities to groups, while “Agent-based” models apply them to simulated individuals. In either case, though, an underlying structure or mechanism is assumed. And key rate or probabilities are usually estimated from multiple sources – usually involving at least some expert opinion or interpretation.
Judging the performance of empirical or inductive prediction models follows a standard path. At a minimum, we randomly divide our original data into one portion for developing a model and a separate portion for testing or validating it. Before using a prediction model to inform practice or policy, we would often test how well it travels – by testing or validating it in data from a later time or a different place. So far, our MHRN models predicting individual-level suicidal behavior have held up well in all of those tests.
That empirical validation process is usually neither feasible nor reasonable with mechanistic or deductive models, especially in the case of an emerging pandemic. If our observations are countries rather than people, we lack the sample size to divide the world into a model development sample and a validation sample. And it makes no sense to validate COVID-19 predictions based on how well they travel across time or place. We already know that key factors driving the pandemic vary widely over time and place. We could wait until the fall to see which COVID-19 model made the best predictions for the summer, but that answer will arrive too late to be useful. Because we lack the data to judge model performance, the competition can resemble a beauty contest.
I am usually skeptical of mechanistic or deductive models. Assumed mechanisms are often too simple. In April, some reassuring models used Farr’s Law to predict that COVID-19 would disappear as quickly as it erupted. Unfortunately, COVID-19 didn’t follow that law. Even when presumed mechanisms are correct, estimates of key rates or probabilities in mechanistic models often depend on expert opinion rather than data. Small differences in those estimates can lead to marked differences in final results. In predicting the future of the COVID-19 pandemic, small differences in expectations regarding reproduction number or case fatality rate lead to dramatic differences in expected morbidity and mortality. When we have the necessary data, I’d rather remove mechanistic assumptions and expert opinion estimates from the equation.
But we sometimes lack the data necessary to develop empirical or inductive models – especially when predicting the future of an evolving epidemic. So we will have to live with uncertainty – and with vigorous arguments about the performance of competing models. Rather than trying to judge which COVID-19 model performs best, I’ll stick to what I know I need to do: avoid crowds (especially indoors), wash my hands, and wear my mask!
Greg Simon