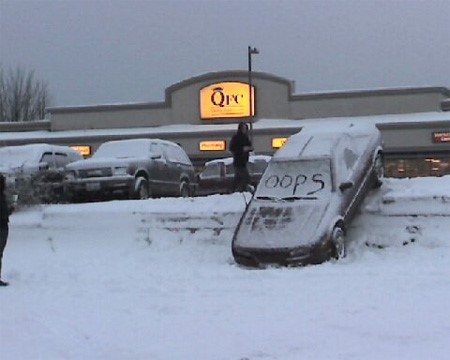
Seattle had one of its rare snowy days last week. Seeing cars slide sideways down our hills reminded me that friction is sometimes our friend.
The modern information economy is all about eliminating data friction. Extracting, combining, and analyzing all types of data get faster and cheaper by the day. As data friction disappears, it seems that nearly any information about nearly anyone is available for purchase somewhere. Since Google controls my phone, Google can sell the information that I go backcountry skiing before sunrise and follow Basset Hounds on Instagram. That doesn’t trouble me. I’m not sure if backcountry-and-Bassets places me in any marketing sub-demographic. But I’m sure my phone knows things about me that I would not want Google to sell. And some people might consider the backcountry-and-Bassets combination to be sensitive information.
Our research group has had some interesting discussions about whether our models to predict suicidal behavior would be even more accurate if we included information from credit agencies. There is no technical or regulatory friction stopping us – or even slowing us down. Credit agency data are readily available for purchase. I suspect many large health systems already use credit or financial data for business purposes. Linking those financial data to health system records is a minor technical task. Machine learning tools don’t care where the data came from, they just find the best predictors. I would expect that information regarding debt, bankruptcy, job loss, and housing instability would help to identify people at higher risk for suicide attempt or suicide death. Whether credit data would improve prediction accuracy is an empirical question, and empirical questions are answered by data. Whether we should use credit data at all is not an empirical question. Even if linking medical records to credit agency data could significantly improve our prediction of suicidal behavior, we may decide that we shouldn’t find out. As data friction disappears, our ethical choices become more consequential.
I think that recent discussions in the medical literature and lay press about the perils of artificial intelligence in health care have mixed two different concerns. One concern is that faulty data or inappropriate methods might lead to inaccurate or biased prediction or classification. The other is that prediction or classification is intrusive and undermines a fundamental right to privacy. We would address the former concern by trying to improve the quality of our data and the sophistication of our prediction models – by further reducing friction. But we would address the latter by slowing down or stopping altogether.
Driving on streets free of friction demands close attention. If I can’t count on friction to save me from risky driving decisions, I’ll need to look far ahead and anticipate any need to slow down, stop, or change direction.
Greg Simon