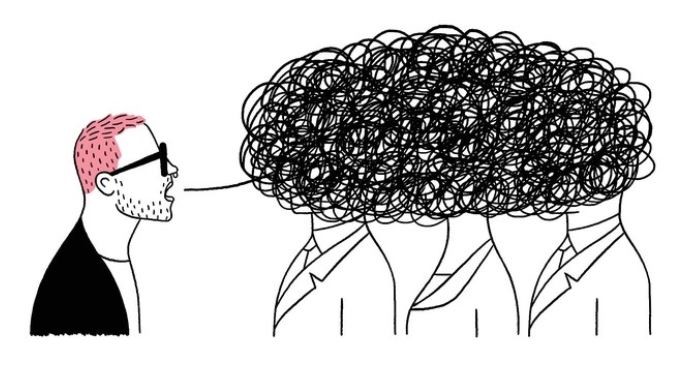
My wife handed me a recent issue of The New Yorker and recommended the Shouts and Murmurs column. It parodied a whistle-blowing data scientist testifying before Parliament about modern analytic methods. He grows increasingly frustrated as legislators can’t follow his explanations of eigenvectors and dimensionality reduction.
At first read, I didn’t think it was very funny. Then I realized: If you don’t think Shouts and Murmurs is very funny, then it’s probably about you.
Much of our work is dimensionality reduction, even if we don’t call it that. Models to predict suicidal behavior or outcomes of depression treatment are all about reducing tens or hundreds of characteristics to a single probability. Old-fashioned regression models are also a tool for dimensionality reduction; they just typically consider a smaller number of dimensions. Moving from the statistical to the clinical, diagnoses are also dimensionality reducers. For example, DSM criteria for the diagnosis of a depressive disorder take nine diverse characteristics and summarize them as a single classification. Going farther back in our psychological history, Sigmund Freud’s The Interpretation of Dreams was all about reducing dimensionality – explaining the wild diversity of human mental life in terms of a few basic instincts and countervailing defenses.
So it should not surprise us that objections to modern statistical dimensionality reduction echo older objections to diagnostic or psychoanalytic dimensionality reduction. Human beings are not one-dimensional. Or even two-dimensional. Our wide experience of joy, pain, hope, loneliness, passion, fear, and tenderness just cannot be contained in a single mathematical model or even a few instinctual drives.
Still, I put more stock in statistical dimensionality reduction than diagnostic or psychoanalytic dimensionality reduction. To generalize, I’m skeptical about any reductionism determined by human “experts”. When we humans try to simplify complex reality, we too often over-reach. Statistical dimensionality reduction tends to be more realistic, or even humble. Our mathematical models only claim to explain or predict a single dependent variable. A statistical model to predict likelihood of psychiatric hospitalization makes no claims to predict success in relationships or finding meaning in life. Predicting risk of hospitalization is practically useful – in a one-dimensional way. Let’s leave it at that.
You may be about to ask, “Then what is an eigenvector?” I’ll pass on that one.
Greg Simon