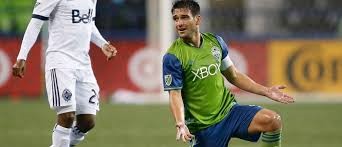
When Nico Lodeiro falls down in the penalty area, I hold my breath waiting for the referee’s call. Was it really a foul – or just Nico simulating a foul? The stakes are high. If the referee calls it a foul, it’s a penalty kick and likely goal for my Seattle Sounders. If she calls it a simulation or a dive, then it’s a yellow card warning for Nico. After the call, we all watch the slow-motion replay. I used to be surprised at how often the refs got it right until a referee friend of mine explained what the refs are looking for.
It goes back to Newton’s first law from high school physics: a body in motion will remain in motion unless acted on by an external force. If Nico was actually shoved, then his speed or direction will change. If instead he just threw himself to the ground, then his overall momentum won’t change. If he throws his shoulders in one direction to simulate a foul, then his arms or his hips have to move in the other direction. With no external force, his center of gravity continues on the same path. That’s how the experienced referee distinguishes a foul from a dive.
When we use health records data to infer causation (e.g. Did a specific treatment make things better or worse?), we also depend on Newton’s first law. We believe that an individual’s mental health path will maintain the same momentum unless it’s acted on by some external force. That force could be a positive or negative effect of treatment or some positive or negative life event. We try to predict an individual’s mental health path or trajectory so we can detect a change in speed or direction.
But mental health is more complicated than high school physics. Any individual’s center of gravity is harder to pin down. Our measures of mental health speed and direction are much less accurate. Mental health paths are often not straight lines. And many forces are often acting at the same time. So we would be foolish to infer causation from a single case. For any individual, our calls about causal inference will never be as accurate as a good soccer referee. But we hope to make better calls by improving the accuracy of our measurement and by aggregating data across many, many cases.
We’ve been surprised by some of our recent efforts to predict mental health paths or trajectories. Risk of suicide attempt or suicide death after a mental health visit is surprisingly predictable. So we expect to be able to detect the effects of external forces – like positive or negative effects of treatments – on risk of suicide attempt. In contrast, probability of significant improvement after starting psychotherapy for depression is surprisingly unpredictable. If we hope to detect effects of more or less effective psychotherapy, we’ll have to first make better predictions. Going back to the soccer story, we would need a clearer view of exactly how Nico was moving before he fell.
Greg Simon