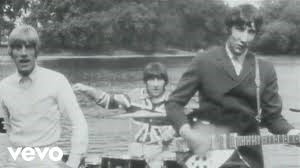
If you’re my age, “The Kids Are Alright” names a song by The Who from their 1966 debut album, My Generation. If you’re a generation younger, it names a movie from 2010. If you’re even younger, it names a TV series that debuted just last year. What goes around comes around – especially our worry that the kids are not alright.
That worry often centers on how the kids’ latest entertainment is damaging their mental health. In the 1940s, the villain was comic books. Prominent psychiatrist Fredric Wertham testified to the US Congress that “I think Hitler was a beginner compared to the comic book industry.” In the 1960s and 1970s, it was the kids’ music – especially songs like “My Generation” with lyrics like “I hope I die before I get old.” In the 2010s, our concern is about screen time – especially time kids spend on social media and gaming. Last year, the World Health Organization added Gaming Disorder to the addictions chapter of the International Classification of Diseases.
Evidence regarding screen time and kids’ mental health is mixed – both in quality and conclusions. Some have pointed to population increases in screen time alongside population increases in suicidal behavior, but that population-level correlation may just be an example of the ecological fallacy. Some large community surveys have found an individual-level correlation between self-reported screen time and poorer mental health. But that correlation could mean that increased screen time is a cause of poorer mental health, a consequence of it, or just a coincidence. A team of Oxford researchers used data from actual logs of screen time and used more sophisticated analytic methods to account for other differences between heavier and lighter device users. Those stronger methods found minimal or no correlation between screen time and teens’ mental health.
A definitive answer would require a true experiment – randomly assigning thousands of kids to higher or lower levels of screen time. That will certainly never happen. If we are left with non-experimental or observational research, then we should ask a series of questions: Did the researchers declare or register their questions and analyses in advance? How representative were those included in the research? How accurately were screen time and mental health outcomes measured? How well could the researchers measure and account for confounders (other differences between frequent and less frequent device users that might account for mental health differences)? We should also recall a general bias in mental health or social science research: Findings that raise alarm or confirm widely-held beliefs are more likely to be published and publicized.
Nevertheless, increasing rates of youth suicide are a well-established fact. And community surveys do consistently show rising levels of distress and suicidal ideation among adolescents. And I don’t think we’ve seen any evidence that time on social media or gaming has a positive effect on mental health or suicide risk.
The evidence to date raises questions but doesn’t give clear answers. For now, I’ll remain cautious – both in what I’d recommend about screen time and what I’d say about the data behind any recommendation. It’s certainly prudent to recommend limits on screen time. But it’s not prudent to claim much certainty about cause and effect. Given the evidence we have, any headline-grabbing alarms would not be prudent. I’d prefer not to be remembered as the Dr. Frederic Wertham of my generation.
Greg Simon