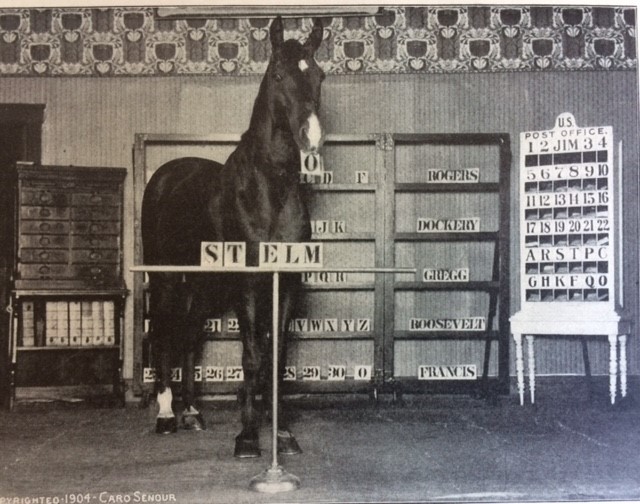
Clever Hans, the Calculating Horse, was a sensation of the early 1900s. He appeared to be able to count, spell, and solve math problems – including fractions! Only after careful investigation did everyone learn that Hans was just responding to unconscious nonverbal cues from his trainer. Hans couldn’t actually calculate, but he could sense the answer his trainer was hoping for.
But even if Hans could actually do arithmetic, a true calculating horse would still be no more than a novelty act. Even in the early 1900s, we had much more accurate tools for calculating. And we had (and still have) much more impressive and useful work for horses.
Some applications of machine learning in mental health seem (at least to me) similar to a performance by Clever Hans. They may involve “predicting” something we already believe and want to have confirmed. Or they may develop and apply a complex tool when existing simple tools are more than adequate. We can use big data and neural networks to mine social media posts or search engine queries and discover that depression increases in the winter. But that’s not news – at least for those of us living in Seattle in November.
As with calculating horses, we also have more impressive and useful work for machine learning tools to accomplish. I think that our work on prediction of suicidal behavior following outpatient mental health visits is a good example. Traditional clinical assessments are hardly better than chance. Simple questionnaires are certainly helpful, but still lack in sensitivity and ability to identify very high risk. There’s clearly an unmet need. Prediction models built from hundreds of candidate predictors can accurately identify patients who are at high or very high near-term risk for suicidal behavior. Our health systems have decided that those predictions are accurate enough to prompt specific clinical actions.
I think we could list some general characteristics of problems suitable for machine learning tools. In practical terms, we are interested in problems that are both difficult (i.e. we can’t predict well now) and important (i.e. we can do something useful with the prediction). In technical terms, prediction models will probably be most useful when the outcome is relatively rare, the true predictors are diverse, and the available data include many potential indicators of those true predictors. In those situations, complex models will usually outperform simple decision rules fit for human (or equine) calculation.
Horses are capable of many marvelous things. I’d pay to see performances by racing horses or jumping horses. And especially mini-horses. But counting horses or spelling horses….not so much.
Greg Simon