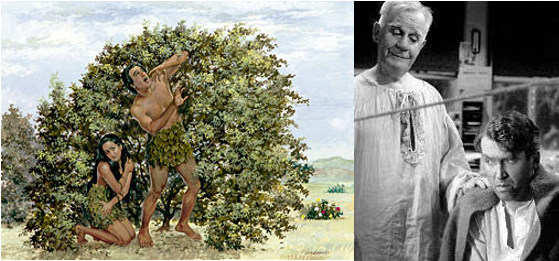
As our health systems prepare to implement statistical models predicting risk of suicidal behavior, we’ve certainly heard concerns about how that information could be misused. Well-intentioned outreach programs could stray into being intrusive or even coercive. Information about risk of self-harm or overdose could be misused in decisions about employment or life insurance coverage. But some concerns about predictions from health records are unrelated to specific consequences like inappropriate disclosure or intrusive outreach. Instead, there is a fundamental concern about being observed or exposed. When we reach out to people based on risk information in medical records, some are grateful for our concern, and some are offended that we know about such sensitive things. And some people have both reactions at the same time. The response is along the lines of “I appreciate that you care, but it’s wrong that you know.” It’s being observed or known that’s the problem, even if nothing is ever said or done about it.
Our instinctual ambivalence about being known or observed was not created by big data or artificial intelligence. In fact, that ambivalence is central to the oldest story that many of us have ever heard: Eve and Adam’s fall from being caringly understood to being shamefully exposed. Both the upside and the downside of being observed are also central to many of our iconic modern stories. The benevolent portrayal of continuous observation and risk prediction is Clarence, the bumbling guardian angel who interrupts George Bailey’s suicide attempt in It’s a Wonderful Life. The contrasting malevolent portrayal of observation and behavioral prediction includes Big Brother in George Orwell’s 1984 and the “precogs” in the 2002 film Minority Report (based on a science fiction short story from the 1950s).
Big data and artificial intelligence do, however, introduce a new paradox: The one who observes us and predicts our behavior is actually just a machine. And that fact could make us feel better or worse. An algorithm to predict suicide attempt or opioid overdose might consider all sorts of sensitive information and stigmatized behaviors to identify people at increased risk. But the machine applying that algorithm would not need to reveal, or even retain, any of that sensitive information. The predicting machine would only need to alert providers that a specific patient is at elevated risk at a specific time. So we might feel reassured that predictive analytics can improve quality and safety while protecting privacy. But the reassurance that “it’s just a machine” may not leave us feeling safe or cared for. Ironically, we may be more likely to welcome the caring attention of Clarence the guardian angel exactly because he is very human – prone to error and unable to keep a secret.
Privacy is complicated, and we often have conflicting feelings about being known or understood. Moreover, our feelings may not match with an “objective” assessment of exposure or risk. But feelings about privacy matter, especially when we hope to maintain the trust of members our health systems serve. We are in for some interesting conversations.